AI Use Cases for Commercial Banking
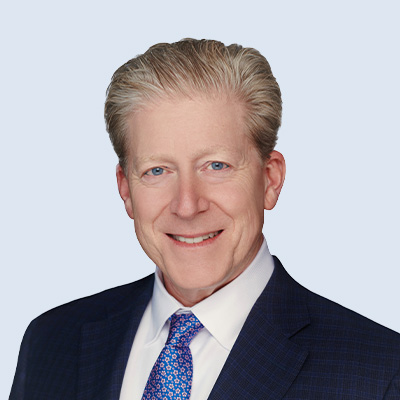
Artificial intelligence (AI) continues to transform the go-to-market landscape today. Whether it’s through increasingly common tools such as chatbots or more advanced machine learning (ML) and generative AI (GenAI) applications, it is no longer a futuristic concept, but rather an everyday reality. As competition intensifies and customer expectations rise, embracing AI is crucial for B2B organizations looking to stay ahead of the curve and drive sustainable growth.
As the financial services industry embraces this digital transformation, AI technologies are becoming integral to banking operations, offering innovative solutions that enhance efficiency and improve customer experience. Many organizations in the commercial banking sector have been able to take learnings from their B2C motions and apply them to their B2B sales, streamlining the adoption and increasing the efficacy of these new technologies.
This article will examine two case studies of AI applications in commercial banking, highlighting both the pain points that led to the investments, as well as the quantifiable ROI associated with these investments.
Case Study #1: Developing a Qualified Pipeline with AI
Following the rise of virtual B2B sales in the wake of the pandemic, a major corporate credit card provider began feeling the effects of the newly and rapidly transforming seller-customer interactions and sales oversight. At that time, the company tech stack was unable to identify incremental sales opportunities within existing accounts, with the organization’s marketing and sales contacts being tracked in siloed systems.
As a result, the company needed an innovative solution that would help sellers unlock the additional revenue potential within their existing accounts, while also making prospective customer conversions more efficient to drive growth.
Approach
As a solution, the credit card provider developed an in-house machine learning algorithm to dynamically route prospects from the marketing to sales pipeline at the most opportune points. To develop the algorithm, the company:
- Increased and integrated available prospect data by investing in first-party data capture to then synthesize contextual/ behavioral data (e.g., website browsing patterns, email engagement), channel interactions and purchase patterns for their existing customers.
- Created business logic for what prospect characteristics and engagement thresholds would inform a range of pipeline actions, from delivering marketing primers to assigning white-glove sales coverage.
- Integrated performance data from similar lead types to continually refine predictions.
Outcome
During the 18-month pilot, the company achieved 1.5-2x higher conversion rate and saw increased incremental spending from leads funneled through the newly AI-enabled pipeline.
Case Study #2: Leveraging AI to Cross-sell and Upsell
As part of its annual planning process, this bank defined a goal of achieving growth in their SMB accounts through analytics and outreach personalization. To do so, the company looked at the recent GenAI boom to inform and develop multiple AI-enabled processes using IBM Watson. The CTO also engaged a team of internal experts across the organization to identify the most meaningful customer data use cases to reduce acquisition and service costs, all while continuing to grow revenue.
Approach
The company deployed a ML product recommender, which aggregates buckets of customer datapoints (e.g., firmographic, transaction, internet browsing) and then runs a variety of ML algorithms (e.g., random forest, decision tree) to place customers in hyper-localized segments. From there, the tool uses collaborative filtering to rank the segments’ likelihood to purchase each product.
This bank combined the product recommender with a ML next best action algorithm that analyzes customer activity (e.g., logged into portal and viewed loan calculator) to determine propensity to buy each product from the recommendation ranking. As a result, the company was able to elevate its existing virtual assistant, which was originally designed to processes and answer simple customer inquiries (e.g., “Was this transaction approved?”). Once it was connected, the AI stack could then prompt the virtual assistant to gauge customer interest in products with the highest AI-generated recommendation score.
Outcome
Because of the initial success of this AI-enabled process, the bank now has a defined goal of driving 30% of its revenue through their AI-enabled chatbot in the next three years.
Future Outlook
The integration of AI in commercial banking can be a transformative force, driving significant improvements in sales processes and customer engagement. Alexander Group case studies illustrate how AI can be leveraged to develop qualified pipelines and enhance cross-selling and upselling strategies for banks looking to stay competitive in the dynamic marketplace.
Through TAM and customer opportunity modeling, pipeline and forecasting, sales productivity benchmarking and other data-driven go-to-market strategies, Alexander Group helps organizations respond to AI disruptions in the market and achieve profitable topline growth.
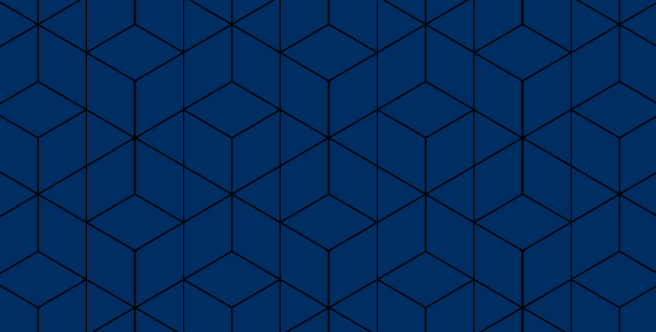
Why Alexander Group?
For more information about AI use cases in commercial banking, please contact an Alexander Group Financial Services practice lead.